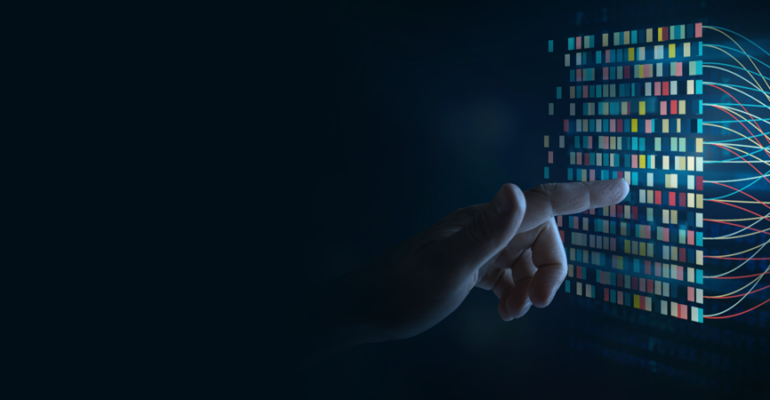
By Alen Alosious
26th Sept, 2024
Introduction
Retrieval Augmented Generation (RAG) has emerged as a game-changing paradigm. As organizations grapple with vast amounts of data and the need for intelligent information retrieval, RAG offers a powerful solution. However, implementing RAG effectively in enterprise settings is far from trivial. Let’s deep dive into the intricacies of RAG, its challenges, and the innovative solutions that are reshaping how businesses leverage their information assets.
1. Understanding RAG
Retrieval Augmented Generation (RAG) represents a significant leap forward in the field of natural language processing and information retrieval. At its core, RAG combines the power of large language models (LLMs) with external knowledge bases to produce more accurate, contextually relevant, and factual responses to user queries.
1.1 The RAG Process
The RAG process typically involves four key phases;
- Indexing: This initial step involves creating a vector index of the data. Vector indexing transforms textual data into numerical representations (vectors) that capture semantic meaning, allowing for efficient similarity searches.
- Query: A user issues a query or question to the system.
- Retrieval: Based on the query, relevant information is retrieved from the indexed data.
- Generation: The retrieved information is fed into a large language model, which generates a response based on both the query and the retrieved context.
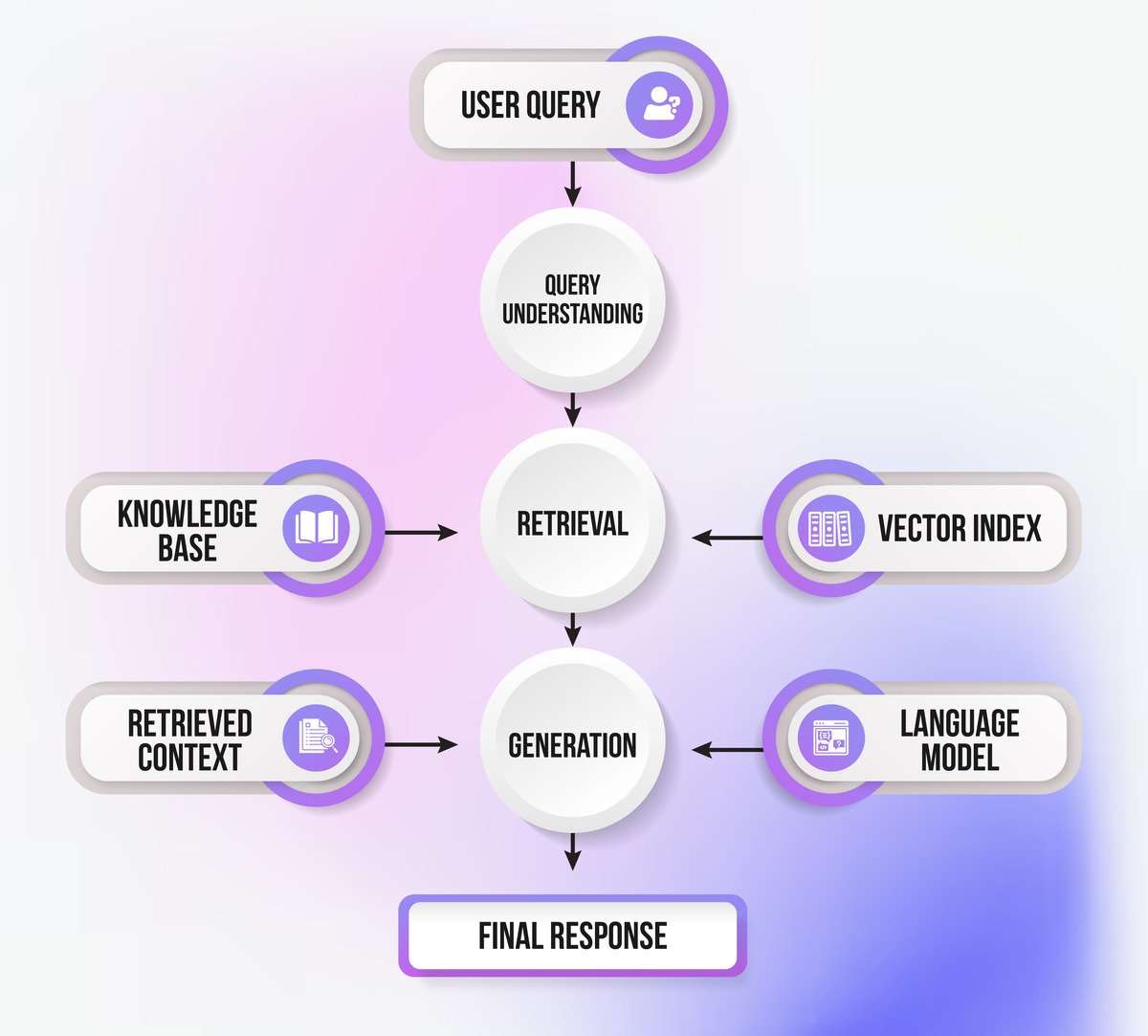
1.2 Why RAG Matters
RAG addresses several limitations of traditional LLMs;
- Factual Accuracy: By grounding responses in external knowledge, RAG reduces hallucinations and improves factual correctness.
- Up-to-date Information: RAG can access the latest information from regularly updated knowledge bases, overcoming the static nature of pre-trained LLMs.
- Domain Specificity: Organizations can tailor RAG systems to their specific domains and knowledge bases, enhancing relevance and accuracy.
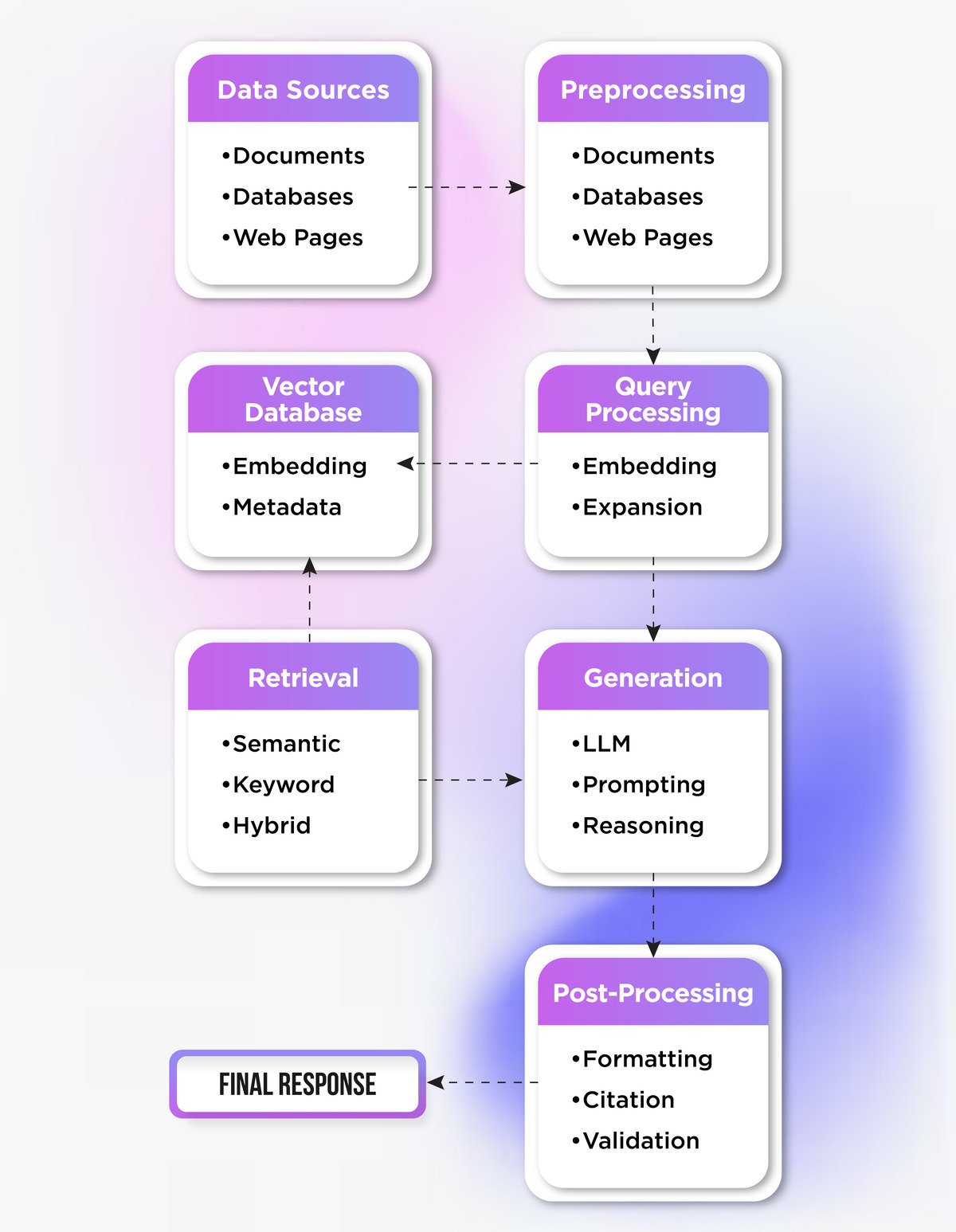
According to a recent McKinsey report, AI technologies like RAG could potentially create $2.6 trillion to $4.4 trillion in annual value across various industries
2. Challenges in Regular RAG
While RAG offers immense potential, implementing it effectively in enterprise environments presents several challenges. Understanding these challenges is crucial for developing robust RAG solutions.
2.1 The Seven Failure Points of Naive RAG
- Missing Content: When the user’s query pertains to information not present in the index, the system may generate hallucinated or incorrect responses.
- Missed Top-Ranked Documents: The answer exists in the document corpus but doesn’t rank high enough in the retrieval process to be included in the context.
- Context Limitations: Relevant documents are retrieved but don’t make it into the limited context window provided to the LLM for generation.
- Extraction Failures: The correct information is present in the context, but the LLM fails to extract or interpret it correctly.
- Format Misalignment: The LLM ignores formatting instructions, producing responses in incorrect formats (e.g., not providing a requested table or list).
- Specificity Issues: The generated answer may be too vague or overly specific, failing to address the query’s intent adequately.
- Incomplete Responses: The system fails to provide a comprehensive answer, omitting crucial details or context.
2.2 Enterprise-Specific Challenges
Beyond these general failure points, enterprises face additional challenges;
- Data Security and Privacy: Ensuring sensitive corporate information is protected while still being accessible for RAG.
- Integration with Existing Systems: Seamlessly incorporating RAG into established enterprise software ecosystems.
- Scalability: Managing RAG performance as data volumes and user queries grow exponentially.
- Regulatory Compliance: Adhering to industry-specific regulations and data governance policies.
A Salesforce study found that 67% of IT leaders cite data security as their top concern when implementing AI solutions
3. TeqPlatform
TeqPlatform a comprehensive solution from Teqfocus that builds upon and enhances AWS’s capabilities, addressing the challenges of implementing RAG in enterprise environments.
3.1 Leveraging AWS Foundation
TeqPlatform utilizes AWS’s robust infrastructure and services as a foundation, including;
- Amazon S3 for scalable storage of source documents
- Amazon Bedrock for access to state-of-the-art language models
- Amazon OpenSearch for efficient vector search capabilities
3.2 TeqPlatform’s Enhanced RAG Pipeline
TeqPlatform extends the basic RAG pipeline with several innovative features;
1. Intelligent Data Ingestion
- Multi-source integration (e.g., S3, web scraping, enterprise databases)
- Advanced document parsing for complex formats (PDFs, nested tables, images with text)
- Automated metadata extraction and tagging
2. Sophisticated Indexing
- Hybrid chunking strategies (fixed, hierarchical, semantic)
- Custom chunking via serverless functions
- Multi-modal indexing for text, images, and structured data
3. Query Understanding and Reformulation
- Intent classification to route queries appropriately
- Query expansion and disambiguation
- Sub-query generation for complex questions
4. Enhanced Retrieval
- Hybrid search combining keyword, semantic, and knowledge graph approaches
- Dynamic context window adjustment based on query complexity
- Relevance feedback mechanisms for continuous improvement
5. Augmented Generation
- Intent classification to route queries appropriately
- Query expansion and disambiguation
- Sub-query generation for complex questions
6. Post-Processing and Presentation
- Answer formatting and structuring based on query intent
- Citation and source tracking for transparency
- Confidence scoring and uncertainty quantification
3.3 Enterprise-Focused Features
TeqPlatform addresses enterprise-specific concerns with;
- Fine-grained access controls and data encryption
- Audit logging and compliance reporting
- Seamless integration with existing enterprise authentication systems