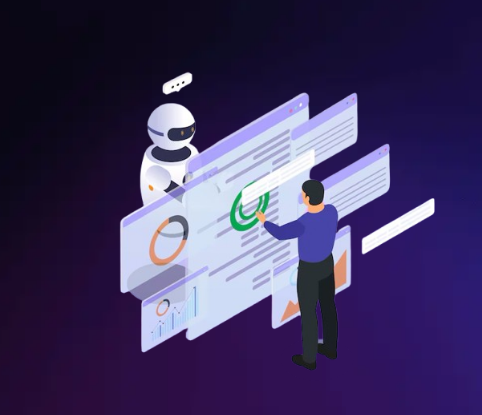
Strategic Data Integration: The Unseen Engine
Behind Scalable AI
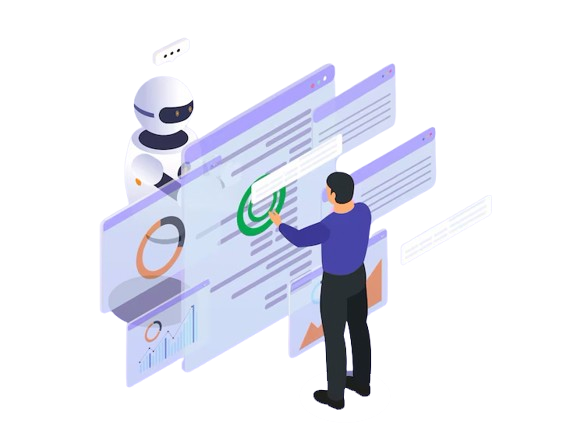
By Avi Kumar & Alen Alosious
14th May, 2025
This isn’t just a pithy quote – it’s a reality check for every organization chasing the promise of enterprise AI. In the first part of our AI Transformation Series, we tackled the foundational problem of data silos. Now, we move to the next essential layer – strategic data integration – because without it, even clean data remains useless for real-time intelligence.
“If your data can’t move, your AI can’t think.”
The Reality: Your Data Exists. But It’s Not Integrated.
Modern enterprises live in a fragmented data landscape – cloud platforms, on-prem servers, CRMs, ERPs, third-party tools, IoT feeds, and API calls. Gartner estimates that the average enterprise uses more than 1,000 cloud services, many of which are disconnected.
The result?
- Data is “available” but not actionable
- Reports are outdated by the time they’re run
- AI pilots are detached from live business signals
- Teams are glueing together insights instead of generating outcomes
And the cost of this disjointedness is massive. According to McKinsey, poor data quality and disconnected systems cost businesses 20–30% of their revenue every year.
Traditional Integration Won’t Cut It Anymore
The old world of integration – manual ETL scripts, batch jobs, brittle point-to-point pipelines – was built for BI dashboards, not for real-time machine learning models or generative agents.
Key problems with traditional approaches;
- High latency: AI use cases demand real-time responsiveness, not overnight batch refreshes.
- High maintenance: Every integration becomes technical debt.
- Poor scalability: Each new data source adds fragility.
- No semantics: Data moves, but without meaning or business context.
This approach may have worked in the past, but it’s now a bottleneck for AI transformation.
The Modern Stack: Real-Time, AI-Centric Integration
Today, forward-looking enterprises are shifting to AI-ready integration platforms that are dynamic, scalable, and intelligent by design.
Let’s dive into two critical players reshaping enterprise integration;
1. MuleSoft – Integration That Speaks Enterprise
MuleSoft provides API-led connectivity across legacy and modern environments. It’s not just middleware – it’s a strategic platform that allows enterprises to unlock siloed data without costly rewrites.
Why MuleSoft matters –
- API-first architecture: Build once, scale across functions and systems.
- Prebuilt connectors: Integrate with Salesforce, SAP, Oracle, AWS, and more.
- Legacy bridging: Expose mainframe and on-prem data to cloud services.
- Governed access: Secure, role-based, auditable data pipelines.
Healthcare Use Case – A large provider unified EHR, claims, and care coordination systems using MuleSoft APIs. This integration enabled real-time AI-generated care plans, improving care outcomes and reducing manual coordination delays.
Explore – Mulesoft
2. Salesforce Data Cloud – From Data Lake to AI Lake
Salesforce Data Cloud (formerly CDP) creates a unified, zero-copy data architecture where AI and automation can operate on live data – not stale exports.
What makes it unique –
- Zero ETL: Query and act on data without duplication.
- Semantic modeling: Business context is embedded directly into data layers.
- Real-time segmentation: Run dynamic actions (e.g., offers, triggers) in milliseconds.
- AI-ready pipeline: Seamlessly feeds LLMs and ML models with trusted data.
- Governance-first: Identity resolution, lineage, and compliance baked in.
Telecom Use Case – A telecom operator connected call center logs, billing, and device telemetry via Data Cloud to predict churn. AI then triggered proactive retention workflows – reducing attrition and improving NPS.
Explore – Salesforce Data Cloud
Integration Unlocks Real-Time AI Use Cases
When integration becomes intelligent and real-time, AI transforms from a pilot to a business growth engine;
AI Use Case |
Enabled by Integration |
Real-time product recommendations |
Unified behavioral + transactional data |
Generative customer agents |
Live data retrieval from multiple apps |
Predictive analytics |
Continuous feeds from sales, ops, and finance |
Personalized journeys |
Dynamic segmentation + context |
Workflow automation |
Cross-platform event triggers |
This is not theory. Leading companies like Unilever, BMW, and Cigna have embedded intelligent data platforms to power AI at scale.
Architecting the Integration Layer: What to Prioritize
An AI-ready integration strategy isn’t just about speed – it’s about trust, context, and reusability.
Here’s what leaders should focus on;
- Reusable APIs – Build once, deploy across use cases
- Event-driven architecture – Ensure real-time responsiveness
- Unified identity graph – Single customer view across systems
- Observability & Monitoring – Track flow and performance metrics
- Governance from Day 1 – Ensure compliance, lineage, and security
Forrester calls this shift the evolution from “data integration” to “data activation” – where integration platforms do more than move data, they contextualize and orchestrate it for action.
From System Sync to Intelligence Layer – The Big Mindset Shift
This is the transformation;
- From connecting tools ➝ to activating decision intelligence
- From moving rows ➝ to triggering outcomes
- From backend plumbing ➝ to enabling real-time enterprise agility
The companies winning the AI race aren’t just investing in models – they’re re-architecting their data and integration strategy for scalability.
Final Word: AI Without Integration is Just a Demo
Every executive wants their organization to be AI-first.
But here’s the truth – You can’t deploy real AI until you fix real data problems. And without the right integration layer, your AI pilots will remain isolated experiments.
Strategic, governed, API-led integration is the engine room of enterprise-wide AI transformation. It’s what turns siloed insights into enterprise intelligence.
Want to explore what your organization needs to get there?
Let’s talk. Schedule a consultation on AI readiness and integration strategy.