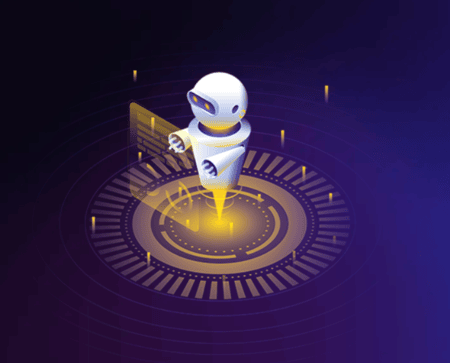
Why AI Strategy Starts with Data, Not the Model
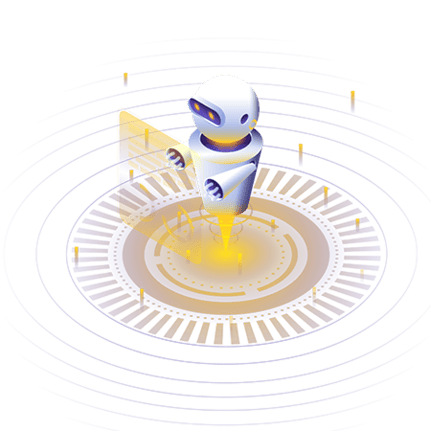
By Avi Kumar & Alen Alosious
07th May, 2025
Start Here, Not There: Why Your AI Strategy Begins with Data, Not the Model
“AI doesn’t fix messy data. It multiplies the mess.”
We’re deep into the AI hype cycle. Boards are demanding it. Business teams are excited. Tech vendors promise magic. But a hard truth is emerging in boardrooms and C-suite strategy sessions across industries:
AI cannot deliver enterprise value if your data foundation is broken.
Before you test-drive generative AI, build predictive analytics, or automate customer experiences, you must ask – Is your data even ready for AI?
Data Silos: The Quiet Killer of Digital Ambition
Let’s call it what it is: siloed data is the silent killer of digital transformation.
Despite years of digital investment, most enterprises still struggle with fragmented data ecosystems. A McKinsey report notes that over 60% of digital transformation efforts stall due to poor data quality and integration.
Even when data resides in the same CRM or cloud platform, it’s often duplicated, ungoverned, or disconnected. The downstream impact?
- Conflicting KPIs across departments
- Manual workflow bottlenecks that cripple automation
- AI hallucinations due to incomplete or inconsistent training data
- Missed revenue opportunities and delayed innovation cycles
Consider the example of a global financial services firm. Their data lived in dozens of disconnected systems across retail, insurance, and wealth management. Attempts to deploy AI-driven personalization failed – until they unified their data across Salesforce, Snowflake, and legacy platforms.
The result? A 40% boost in cross-sell efficiency within 12 months.
You Can’t Innovate on Broken Data
We engage with enterprise leaders weekly who are all-in on AI. But before they can innovate, they’re forced to hit pause and confront fundamental data challenges:
- Where does our mission-critical data actually live?
- Can it be accessed securely and seamlessly across platforms?
- Is it trusted and governed enough to fuel autonomous decisions?
AI is not plug-and-play. It’s data-powered. A recent Forrester report found that 71% of AI leaders cite data issues as the top barrier to scaling AI Forrester.
If your data is inconsistent, isolated, or outdated, no large language model (LLM) or ML algorithm can bridge that gap. Garbage in, hallucinations out.
The Real First Step: Build a Unified Data Foundation
What separates AI leaders from laggards? A data-first approach. They don’t start with the model. They start with the foundation:
- An enterprise-wide data integration strategy
- Clean, governed, accessible data pipelines
- Investment in orchestration tools like MuleSoft and Salesforce Data Cloud
- Cross-functional governance aligning IT, operations, and business units
Organizations like Siemens and Unilever are reaping AI benefits precisely because they began with unified data architecture, not AI pilots. Siemens uses MuleSoft to connect over 600 applications and create a unified view across engineering, operations, and finance – enabling predictive maintenance and AI-enabled quality checks at scale MuleSoft.
The 5-Year Shift: From Siloed Systems to Seamless Data Flow
A new architectural reality is taking hold: the future is not app-first, it’s data-flow-first.
Forward-thinking enterprises are shifting from fragmented systems to integrated ecosystems where data flows freely and securely between edge devices, cloud platforms, and decision engines.
This means;
- Platforms with real-time connectors to cloud, legacy, and SaaS systems
- Moving from heavy ETL pipelines to zero-copy architectures (think Snowflake’s cross-cloud sharing)
- Establishing a single source of truth that powers both analytics and AI
- Enforcing data governance as a prerequisite – not an afterthought – to AI deployment
In a Harvard Business Review article, Bain & Company highlighted that enterprises with fluid data architectures are 5x more likely to achieve AI-at-scale.
Let’s Be Clear: This Isn’t Easy. But It’s Essential.
Fixing fragmented data doesn’t earn you instant headlines. It’s not a splashy launch. But it is the hard work that delivers sustainable value.
Ask any CDO or CIO who’s deployed AI responsibly: the biggest effort wasn’t choosing the model – it was unifying the data and building trust in it.
If you’re serious about AI, don’t start with chatbots or POCs. Start by asking;
Is our data architecture truly ready for intelligent automation and analytics?
Because when the data flows, everything downstream improves – from customer experience to operational efficiency to competitive agility.
Ready to Accelerate?
If you’re evaluating enterprise AI, now is the time to pressure test your data foundation.
Let’s talk – Book a strategy session to assess your AI readiness or co-create a data-first roadmap with our experts.